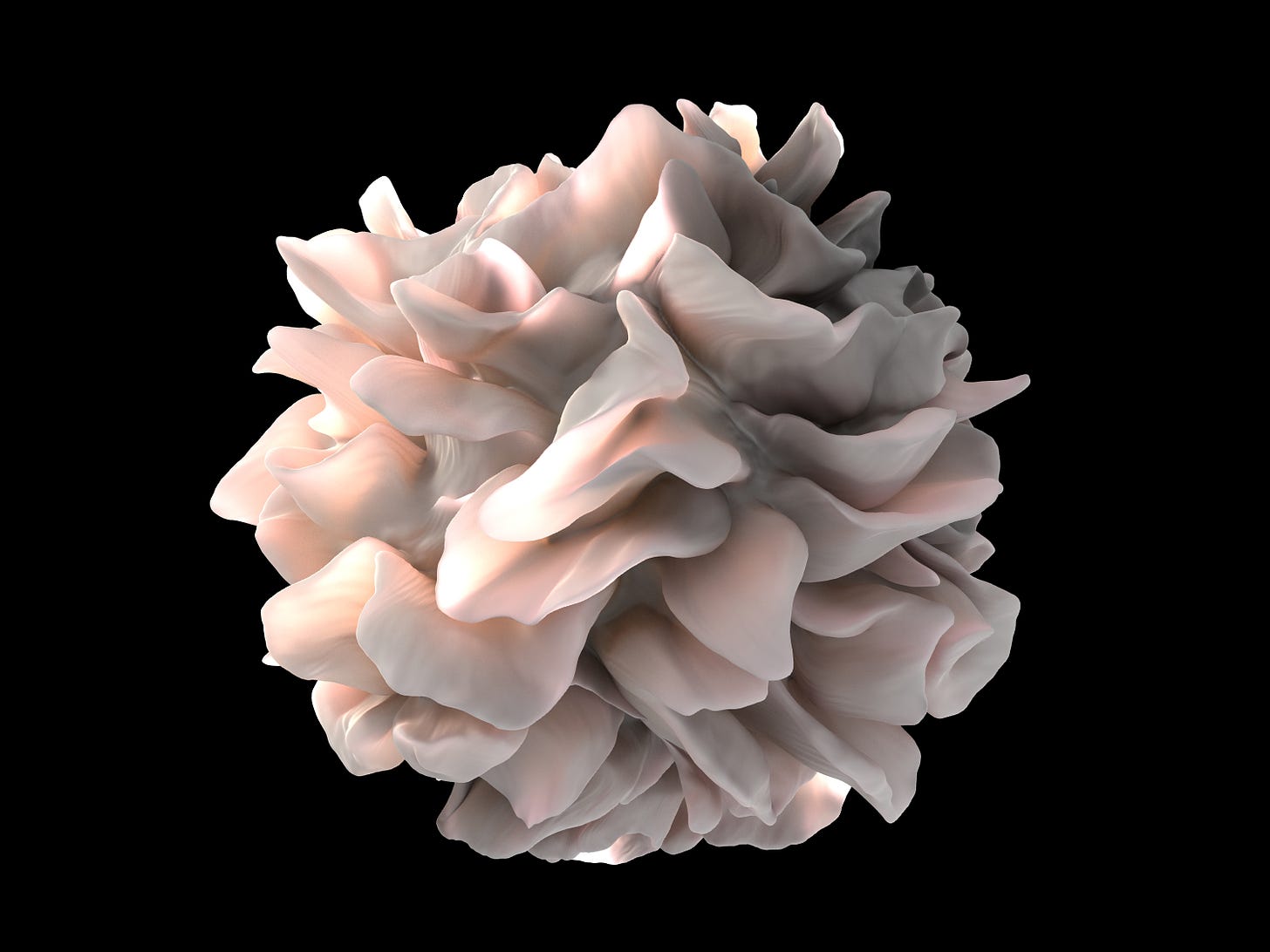
Playing Where’s Waldo with only a description of what Waldo looks like would be awful. Your description would need to have a lot of detail to distinguish Waldo from other people wearing stripes or glasses in the image. This would be a no brainer if he had a measurable feature like height that could distinguish him from the crowd . But that’s unlikely -- and honestly whipping out a ruler to play a game doesn't sound very fun.
Strangely, biologists often play this game on a microscopic scale. They sift through a large number of cells to find and isolate a subset for further study -- some Waldos. Quantitative measurements have been generated, akin to determining Waldo’s height, that allow them to more easily find a cell that fits a specific criteria. But sometimes the best way to tell the difference between one cell and another is qualitative -- simple differences between how they look under the microscope.
Highly trained specialists can use morphology -- the fancy term for how a cell looks -- to classify the difference between two cells, limiting the skill to a select few and inhibiting its widespread adoption. But this training is currently essential if we want to, for example, spot the differences between a regular lymphocyte and a hairy cell leukemia (as in the picture below). In many cases, we wouldn’t even be able to use the simple quantitative measurements that have become ubiquitous in biotech because there are often no measurable markers to distinguish a diseased cell from a healthy one.
What's worse is that a morphological classification ends there. Even if you do have a specialist to spot the right cell, there are no “cellular tweezers” to grab the cell for further study.
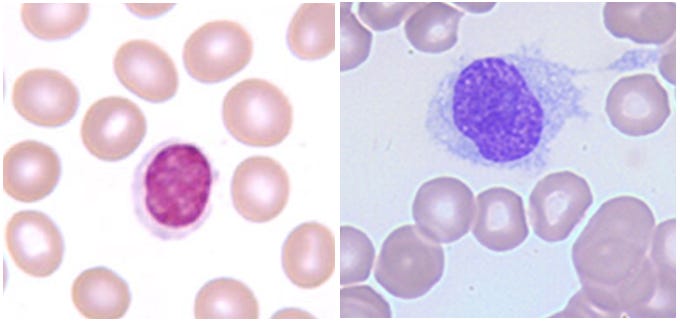
To enable basic, translational, and clinical research at scale, we need a technology that both democratizes cell classification by morphology and leverages visual features to isolate cells.
Deepcell has taken this challenge head on by combining artificial intelligence, microfluidics, and high-throughput imaging to enable anyone to classify and sort cells by morphology. They are the first to use morphology to rapidly and easily define and isolate specific cells. Deepcell has invented a microfluidic platform that enables high-resolution images to be taken of single cells as they flow through the device. These images capture features on the subcellular level, providing visual information that is unavailable to a human eye.
With an unprecedented ability to quickly capture high-quality single cell images, Deepcell is building the largest database of cellular morphologies the world has ever seen -- currently containing nearly 400 million cells and over a billion images. With it, they are creating an ever-growing atlas that connects computer-defined visual features of a cell to functional insights and ultimately to a specific cellular classification.
By using imaging, Deepcell has created a label-free solution to cell sorting and unlocked huge potential for cell biology research. The platform technology uses deep neural networks trained on this data to classify a cell while it's flowing through the device.
The microfluidics are specifically designed to keep the cell nice and happy as it’s imaged and classified. At its output, the device sorts the cell based on its classification to isolate cells with a certain look. Then, researchers can take the morphologically identical cells and perform downstream analyses on them.
Current cell sorting technology relies on quantitative measurements of proteins on the cell; antibodies are used to bind a protein of interest and machines measure whether that protein is present at a specific quantity to decide whether to sort. This is a laborious, slow, and often expensive process that is met with limited results -- we are limited by our knowledge of what proteins to look for and by the number of proteins our machines can read at once. This antibody-based process can also be harsh on cells, often making it an endpoint measurement.
Deepcell’s technology overcomes these challenges. Using morphology as the sorting heuristic, the cell classification becomes unbiased. No longer are we limited to sorting on the protein markers that we think define a class of cells, breaking free of our knowledge gaps, enabling more robust science, and leading to a hypothesis-free analysis. By not defining a cell population to look for beforehand -- an obligatory step with antibody methods -- cell classes can emerge that may have gone previously undiscovered. Finally, Deepcell’s platform was designed around the idea that scientists need the cells alive at the end, so the cells are not hurt by the process.
Deepcell’s platform makes it ideal for modernizing routine procedures. For example, flowing patient samples like tumor biopsies or blood through the device gives unprecedented views into its cellular constituents:
In the time it takes to get a white blood cell count, Deepcell gives you the same datapoint and tells you whether any of those cells look cancerous, infected, or nutritionally deficient.
Traditional medicine uses the morphology of a cell to confirm a disease diagnosis, but Deepcell’s speed and tractability runs those same tests as a screen, finding issues before they become a problem.
While doctors are busy carefully slicing, staining, and observing tumor biopsies, Deepcell has already given the patient a full view of the specific immune cells, support cells, and cancer cells present in the same sample.
As the world's sequencing companies fight to find tumor biomarkers that come close to telling them about the disease’s traits, Deepcell analyzes the same tumor using a platform based on the fact that morphology is the disease trait they were trying to sequence all along.
The platform technology also enables processes that we have only dreamed of. Deepcell can isolate extremely rare populations, capturing subsets that make up 0.0000001% of the population. This means they can capture cancer cells in the blood as a screening tool, catching the onset of a tumor well before symptoms appear and massively improving the chance of a patient’s survival. With this specificity, they could even capture fetal cells circulating in the mother, enabling non-invasive prenatal testing.
Deepcell envisions a living machine improving over time as the community around it grows. Unlike the research and medical devices currently in use, the morphological data that this platform makes is continuously used to train the AI that makes sorting decisions. This creates an ecosystem that consistently improves over time, catching finer and finer differences to stratify and isolate cells on. There are applications that we have yet to even imagine for this technology. Ultimately, Deepcell will empower scientists and clinicians to use their novel platform and sophisticated AI to build a better understanding of biology and improve human health.
They have the team to do it. Co-founder and CEO Maddison Masaeli studied Electrical Engineering undergrad in her native Iran, got her PhD in Biomedical Engineering from UCLA, and did her postdoc at Stanford University in Computational Genomics, where she developed the core Deepcell technology. Co-founder and CTO Mahyar Salek got his PhD in Computer Science from USC, previously founded one of the first companies to ship a deep-learning based solution, and was working in AI at Google before leaving to found Deepcell. Scientific co-founder Euan Ashley is a Professor of Medicine and Genetics at Stanford University.
At Fifty Years, our sweet spot is supporting founders at the earliest stages who are building deep tech companies that can generate huge financial outcomes and create massive positive impact.
Deep Tech: Maddison, Mahyar, Euan, and the rest of the Deepcell team have dedicated their scientific careers to generating a first in class amalgam of microfluidics, imaging technology, and computer vision to give an unprecedented view into a cell’s biology.
$1B yearly revenue potential: By overcoming the limitations of current sorting methods that are ubiquitously used in biology, biotech, pharma, and clinical practice, Deepcell is poised to become the de facto application to classify and sort cells in all its embodiments.
Massive positive societal impact: From bench to bedside, Deepcell’s technology reduces the labor and cost that go into cell classification and isolation while making it more robust and improving its chances of finding unexpected results. This combination creates massive potential to improve patient outcomes, either by directly improving clinical care or by indirectly enabling new medicines to be generated.
Deepcell is an example of how excellent science can create extraordinary impact through entrepreneurship. Inspired by their vision for a better future, Fifty Years was proud to partner with Deepcell in their seed round led by our friends at Andreessen Horowitz and to deepen the partnership in their Series A, lead by Bow Capital, alongside Andreessen Horowitz, DCVC, and Stanford University. At Fifty Years, helping great scientists become great entrepreneurs is our jam, and we’re looking forward to helping Maddison, Mahyar, and Euan make real their vision of redefining biology, one cell at a time.