Valence Discovery
Fifty Years partners with Valence to design better drugs with AI-based generative chemistry
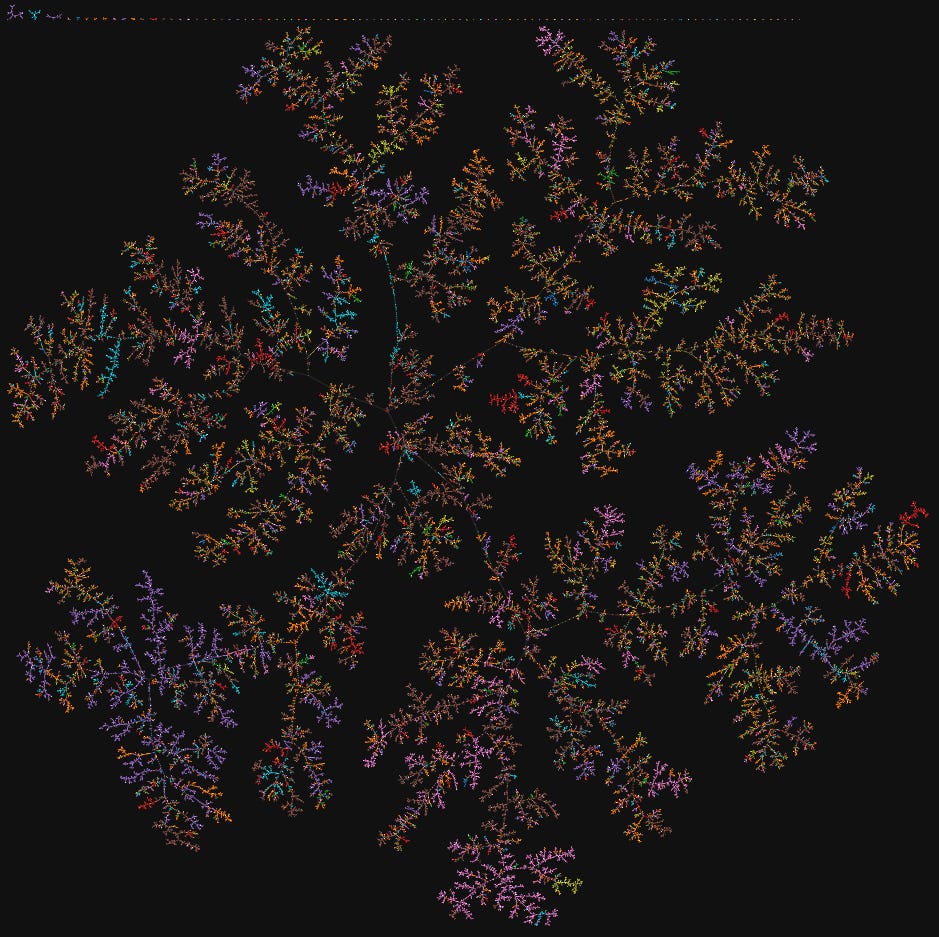
Goofy knows a shocking amount about drug discovery. In this short (watch it!) Goofy goes through endless keys on a keyring to find the right one to unlock a door. In the early stages of drug discovery, pharma’s keyring – their arsenal of molecules – is full of keys that unlock nothing. Sometimes their keyring looks like Goofy’s, where they know what the keys do but they clearly aren’t useful for the door they’re trying to open. Other times they might see a door but can’t find the keyhole. Unfortunately, Mickey’s sentiment is true for many drug discovery endeavors: searching for the right key leaves us disappointed and confused, especially when none of the molecules in the existing arsenal work. The complexity of this problem is a big reason why drug discovery is such a complex and expensive endeavor, and why it takes an average of $1B to get a new drug to market.
Yet, almost every drug on the market was found in this cartoonish way. Pharma spends huge amounts of time, people power, money, and material synthesizing chemicals that go into their expansive libraries – the enormous number of keys on the keyring – and then more time and money testing these experimentally to see if they work. The libraries they use are optimized to create a large diversity of chemical structures and physical properties in order to represent as much “chemical space” as possible. These ever-growing and massive chemical libraries are carefully stored in large warehouses of well-plates (like the one shown below) awaiting the moment pharma finds a new door. For example, Bayer’s library has around 4 million different compounds it can use to screen against new targets.
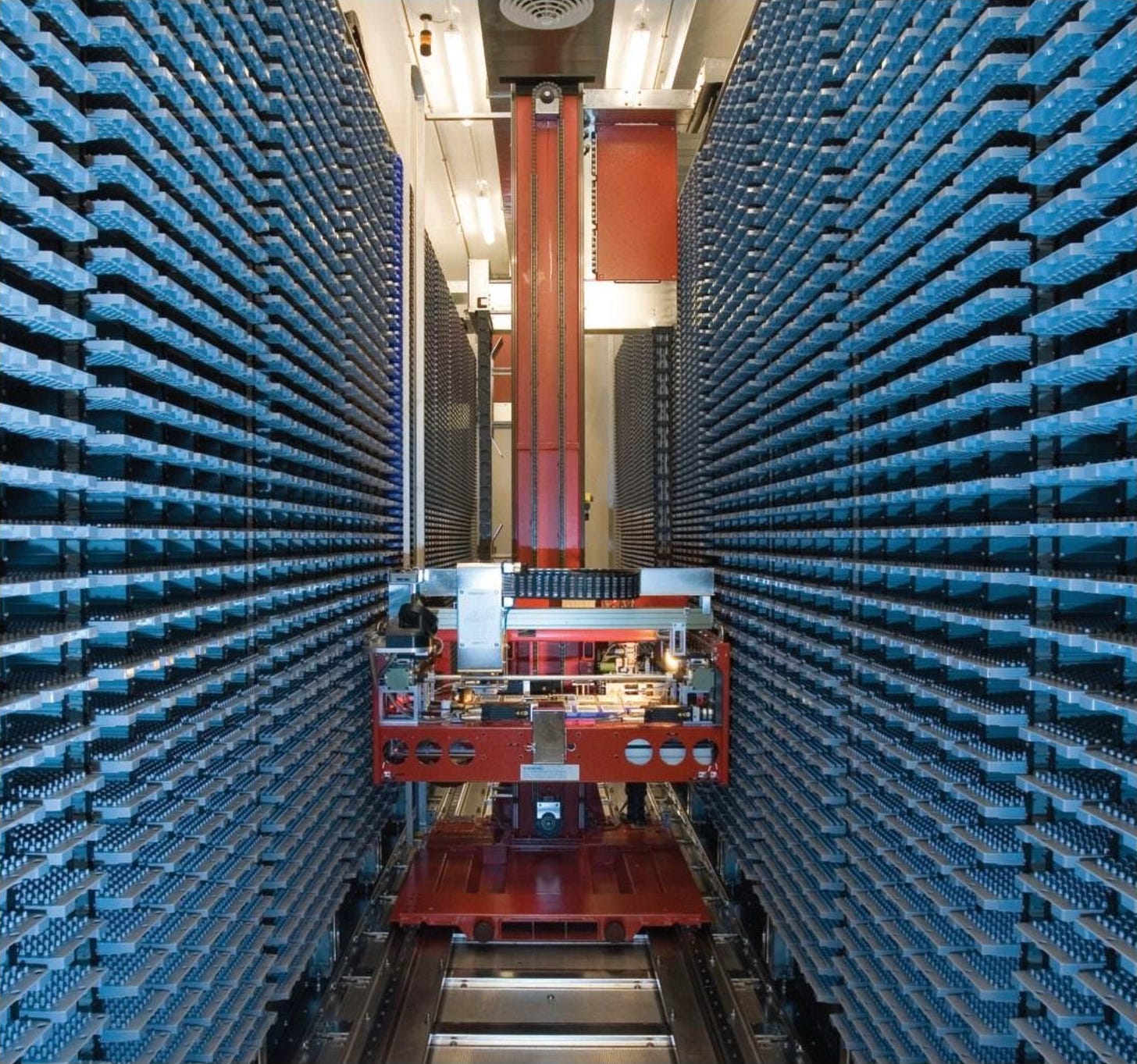
With molecules in hand and chemists working around the clock to expand the library, pharma must look for high quality targets – in our Goofy analogy, the doors that open to effective treatments. Targets are usually proteins that a disease-causing cell expresses. For cancer, the target could be a receptor that helps the cancer grow. For a metabolic disease, it could be a mutated enzyme that no longer functions properly.
Target and chemical library in hand, pharma begins attacking the problem like in the cartoon. They create cellular models of disease that can tell scientists when the target has been disrupted (i.e. the door unlocked) and grow the cells in super tiny well-plates. Using robots, the molecules are added to the cells, one molecule to one well, and measured one-by-one to see if the molecular perturbation produced the desired effect at the target. It’s a very brute force process and in the best of cases only 1 out of 1000 of the molecules may be hits that do anything interesting. In the worst cases, against the most challenging targets, they might turn up empty handed.
To complete the discovery process before testing in living organisms, the most promising hits (known as leads) undergo further chemical optimization, called lead optimization, that aims to improve their properties without compromising their ability to hit the target. For example, by adding different chemical subgroups onto each hit, chemists can enhance the molecules by making them more “drug-like” – changing properties such as solubility, likelihood of being metabolized, and molecular weight – without sacrificing potency at the target.
The search problem pharma undergoes in finding hits, iteratively turning hits to leads, and optimizing the leads experimentally is extremely laborious and resource intensive. Thankfully, we now live in a time where search, design, and optimization problems can be solved in silico. We’ve long hoped to leverage our knowledge of chemistry, biology, and computation to reduce the earliest parts of drug discovery into a computational problem. Using the latest deep learning tools, the vast amount of data that pharma and academia has created can now feed predictions to accelerate the process, reduce the failure rate, and unlock targets that may be difficult or impossible to drug using conventional approaches.
Deep learning typically requires extremely large amounts of data to kick off the learning process. There have been great companies founded over the last decade aimed at just this problem – generating huge amounts of quality biological data with robotics and high throughput techniques to feed into machine learning algorithms in order to improve the drug discovery process. The issue is the sheer amount of data needed.
The space of potential chemicals alone is estimated to be around 10⁶⁰. The combinations of potential chemicals and thousands of potential targets is over 10⁶⁶ – that’s more than the number of stars in the universe, by a lot. Existing datasets and deep learning algorithms can help optimize single molecules, or clusters of them, but it’s like exploring one moon of one planet orbiting a single star. It’s effective in understanding that moon, but it tells you next to nothing about other moons in the universe. The traditional data-heavy model of AI drug discovery falls short when you realize 90% of potential chemistries and 97% of targets have no data! Ideally we’d be able to use AI to better understand these unexplored spaces without the need to spin up hundreds of millions of time consuming and expensive biological experiments.
Enter Valence Discovery. Rather than trying to adapt drug discovery to meet the constraints of deep learning, Valence is adapting deep learning to the constraints of drug discovery. Given the sparsity of data available and the intractability of broadly exploring the drug-target space, Valence realized that drug discovery is a “low data problem,” not a “big data problem”.
Valence is creating machine learning systems that enable them to design vastly better molecules with much less (or even no) data, against some of the pharmaceutical industry’s most challenging and high-value targets. By rethinking the way deep learning is applied, they have pioneered multiple approaches that together solve drug discovery’s data problem.
They start by tackling featurization – the way a molecule is represented in an ML model. Featurization attempts to distill the essence of each molecule such that a computer can understand its properties. While this step is essential to translating the physical to the digital, the quality of that translation can have profound effects on how successful the algorithm is at predicting drug efficacy and other key properties. Valence has developed best-in-class methods for molecular featurization. For example, Valence can encode 3D properties directly from 2D structure and better capture key molecular interactions that increase the accuracy of their predictions. These featurization approaches allow Valence to predict drug efficacy with fewer molecularly similar examples, which is especially important for novel or challenging disease targets. The flexibility of these approaches also enables Valence to look beyond classical small molecule chemistry into degraders, molecular glues, macrocycles, and more.
Building on this featurization, Valence leverages their founding team’s pioneering research in few-shot learning against novel targets with limited (or no) existing data. This approach allows their algorithms to extrapolate far beyond the training data – much farther than they ever could with traditional methods. With it, Valence reaches into the vast regions of unexplored chemical and target space that no one else can, finding more potent hits quicker than ever. Even in situations with extremely limited quantities of data, Valence has already shown that they can predict key drug parameters such as potency, selectivity, and toxicity with a level of precision that matches experimental results.
Valence has also innovated on physics-based approaches to simulate how drugs generated by the computer (but never actually synthesized by a chemist) bind to a target of interest. Combining this with state-of-the-art optimization techniques, Valence designs novel hits and improves their drug attributes entirely in silico, bypassing the need for the huge amount of iterative experimentation that can be time-consuming, noisy, and expensive. The power of this approach is already apparent – Valence was able to design a better drug in 2 weeks than pharma could in 2 years (see below).
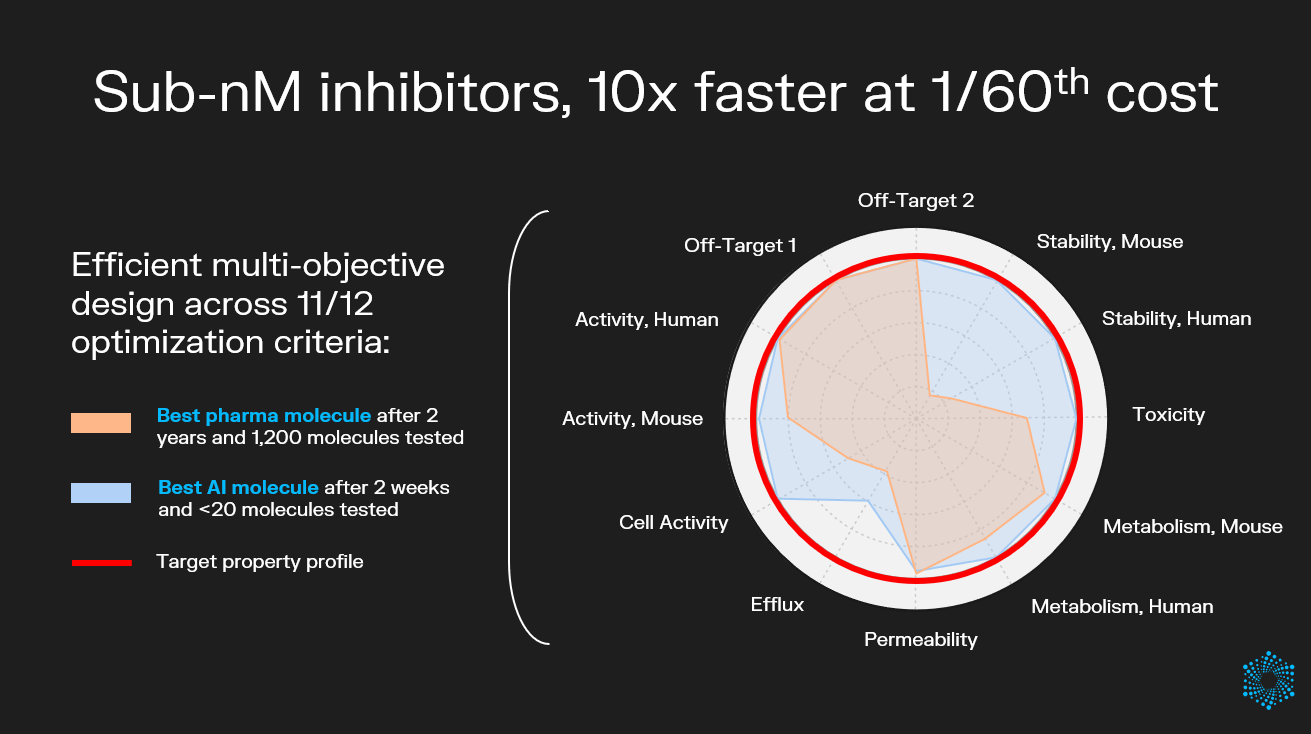
The last piece of Valence’s approach solves the final major challenge in AI-driven drug discovery: can chemists even make the molecule the algorithm designs?
Traditional deep learning approaches often suggest molecules that might hit the desired target but are impossible to manufacture. To ensure they can translate the digital asset to a physical one, Valence created a method that takes a library of molecules that have already been synthesized and uses features of these accessible drugs to train the computer on which molecular additions, building blocks, and chemical reactions are acceptable. It’s like watching chess: after a few games, you can understand how each piece is allowed to move. Once you know how the pieces move, you can begin to imagine what your opponent might do in the future, and start thinking a couple steps ahead. Valence’s method does the same thing, so that when a suggested hit pops out of the algorithm, chemists know they can actually make the molecule.
The most exciting part: it’s already working! Valence’s platform – used by pharmaceutical and biotech companies including Charles River Laboratories, Repare Therapeutics, and Servier – has enabled rapid design of novel chemical matter against extremely challenging disease targets. Valence has demonstrated impressive commercial traction and is actively deploying its platform across multiple target classes and indications through collaborations with leading biotech and pharmaceutical companies, CROs, and academic centers.
These enormous strides in AI applied to drug hunting are only possible because of the expert team behind Valence. Co-founder and CEO Daniel Cohen brings his expertise in cognitive science, computer science, and machine learning from his research as a graduate student at McGill University. Co-founder and COO Therence Bois brings deep experience in molecular and cellular biology from his PhD work in cancer resistance at the Montreal Clinical Research Institute. Co-founder Prudencio Tossou is a PhD in machine learning and a researcher at Mila who pioneered few-shot learning approaches applied to drug discovery. Co-founder Sébastien Giguère is a PhD in machine learning and computational biology as applied to the design of pharmaceutical compounds. The Valence founding team has created an incredible culture driven by science and innovation that keeps them at the forefront of their field.
At Fifty Years, our sweet spot is supporting founders at the earliest stages who are building deep tech companies that can generate huge financial outcomes and create massive positive impact.
Deep tech: The Valence team is pioneering the use of next generation computational technologies custom-built for biology and chemistry, including geometric deep learning, low/no data deep learning, and physics-informed deep learning, across the entire drug discovery pipeline, allowing them to look deeper into drug target space with vastly smaller amounts of training data.
$1B yearly revenue potential: single successful drug can get to $1B+ revenue. Valence is helping create dozens of them across external partnerships with industry-leading discovery organizations as well as an internal pipeline of in-house assets.
Massive positive societal impact: Given the number of disease targets that remain undruggable, and diseases that remain untreatable, Valence’s chemistry engine has the potential to power an entire generation of drug discovery, helping bring new medicines to a broader and more diverse set of patients.
Inspired by their vision for the future of drug discovery, Fifty Years is excited to co-lead Valence’s seed round, along with our friends at Air Street Capital. Amplitude Ventures, Phoenix Venture Partners, and Abcam founder & former CEO Jonathan Milner also participated. We’re looking forward to helping Daniel, Therence, Prudencio, and Seb discover drugs that help billions of people.